Space Force Chief Technology and Innovation Officer Lisa Costa moderated a discussion on “AI Integration” with RB Hooks III, Oracle National Security Group; Kay Sears, Boeing Defense; and Justin Woulfe, Systecon North America, Sept. 21, 2022, at AFA’s Air, Space & Cyber Conference. Watch the video or read the transcript below. This transcript is made possible by the sponsorship of JobsOhio.
If your firewall blocks YouTube, try this link instead.
Lisa Costa:
Good morning everyone, and thanks for joining us today for what I’m certain will be an interesting and informative panel on artificial intelligence and machine learning. I hope that this crowd is indicative of the number of people interested in this topic and interested in developing artificial intelligence and machine learning for the Department of the Air Force because it will take a consortium of partners to move forward.
I’d like to start off by providing a quote by John F. Kennedy, and it was interesting because he was speaking about space at the time that he made this quote, but I think it’s really applicable to the AI environment that we find ourselves in today. So he said, “We set sail on this new sea because there is new knowledge that must be gained and new rights to be won, and they must be won and used for the progress of all people. For space science has no conscience of its own. Whether it will become a force for good or ill, depends on man. And only if the United States occupies a position of preeminence can we help decide whether this new ocean will be a sea of power or a sea of peace or a new terrifying theater of war.”
That’s really quite prescient in terms of just thinking about the space environment that we find ourselves today. But then, add the potential for artificial intelligence and the use of it in space, and there are a lot of challenges I think we find ourselves in. And I’m very excited to have this panel here to discuss some of those challenges and then some of those opportunities.
We’re really sitting at a crossroads. We know AI is critical. We know AI algorithms are being used today in vast quantities of data, and we in the Department of Defense know that it’s our industry partners who are investing significantly in these types of technologies. So we want to partner with you, we need to partner with you, and that is what we are exactly looking to do.
Across the DOD and the Department of the Air Force, we’ve made great strides toward technical modernization. In fact, earlier this year, the National Defense Strategy directed us to establish new acquisition systems, which have the ability to be interoperable with modern and AI ready open architectures. Further, the US has plans to use AI in a variety of space missions while complying with current laws and policies.
We’re leveraging AI to continue building enduring advantages. Some of these advantages exist in current US space applications such as space domain awareness, command and control, missile guidance, automatic target recognition, position navigation and timing applications, and object classification. But we can’t do this alone, and we can’t do it in a vacuum certainly. We have to partner with our allies, industry, academia to solve these problems.
As the chief technology and innovation officer for United States Space Force, I used to be a Special Operations, my team and I look forward to working on these challenges and delivering advanced AI enabled capabilities. With that, I’d like now to introduce our panel and get some conversations started on this very topic. What I’m really excited about is that our panel represents a great mix of strategic, operational, and tactical experiences, and applying AI, and in space in general. So I’m very excited to have that degree of experience here on this panel this afternoon.
So I will introduce Mr. Justin Woulfe, who is the CTO and co-founder of Systecon North America. He has expertise in predictive analytics and systems, as well as logistics and cost optimization. Next is Ms. Kay Sears. She’s the vice president and the general manager of autonomous systems at Boeing Company. And finally, we have Mr. Nick Toscano. He is a machine learning engineer and data scientist at Oracle, with experience in the Department of Defense and Intelligence community. He’s also been a national security analyst and consultant.
So with that, I will hand the mic over to each one of these panelists for a brief intro themselves on their background and what they’re doing in the areas of AI.
Justin Woulfe:
Yeah, thank you. Good morning everyone. Or afternoon, I guess, soon. I’m really bad at this. I’m really passionate about data science, not so good at the whole panel intro side of things. So I decided to actually load several thousand hours of transcripts from things just like this into some NLP algorithms that we use, and let it actually write the intro for me. And actually I was pretty impressed. Sometimes you kind of wonder how this is going to turn out, and it actually turned out pretty good. So here it goes.
So artificial intelligence has prompted us to rethink the very nature of the innovation process. And the pace of innovation in this area is moving fast with 50% of all AI patents being published in just the last five years. Artificial intelligence is being used across the globe to help solve some of our biggest challenges from fighting hunger, to landing reusable rocket boosters, to enabling vehicle commutes with limited human intervention. As we constantly work to cut through the buzzwords and vaporware that drive Gartner’s hype cycle graphs towards the trough of disillusionment, there are some real opportunities for the US Air Force to leverage AI for efficient data analysis, model generation, and to enable better, more defensive analytics that will increase platform readiness.
So look, at its core, if we can do this, imagine what we can do with NLP and reading maintenance records, or enabling better predictive analytics models so that we can really capture future state readiness. I mean, this is some pretty cool stuff. This is real. It’s available today. So, pretty excited about being here. Thank you very much.
Lisa Costa:
And I’m impressed with that auto generation.
Justin Woulfe:
Yeah. Yeah.
Lisa Costa:
Pretty good.
Kay Sears:
Okay. You had a humor AI engine, obviously. My intro, I think, is a little more serious, I guess. I run the autonomous systems part of Boeing, and autonomy is kind of a place where AI has incredible potential, I think, beyond what we can even conceive of right now. We’re focused on introducing autonomy first, and then really evolving the capability with AI and machine learning. But the potential is amazing. If we think about sending autonomous systems out as part of our war fighting initiative, and then those systems can actually perform missions that are performed in different ways today in an increasingly complex environment, I think that, that potential is something that is not only awesome in itself, but is going to be absolutely necessary when we think of the adversary, when we think of the pace of war, when we think of the density of war.
So, we tend to fall back on a simulated environment, which is the tools that we have today. We’re simulating autonomy. We’re simulating the potential for AI applications. But we’re also trying to be very sensitive to the safety around that. How are we going to actually prove and gain the trust of the war fighter in this autonomous and AI enabled environment? And so, I think we just have to be very cautious there. We have to be very thoughtful for how we’re going to apply this AI learning, but the potential is amazing.
And I think in one of the questions, I’ll try to describe more of a crawl, walk, run approach that I think leverages a lot of the digital tools, the autonomy framework, and environment that’s at the core, and then how we gradually add the AI and the machine learning in a safe and predictable way. Because I think that’s going to really make us successful, and it’s going to solve the trust and adoption problem so that we can actually really go to war with these tools and have them perform the way that we’re expecting them to. So I look forward to the discussion. Thank you.
Nick Toscano:
Yeah, everybody. My name’s Nick, and thanks for letting me be here. I’m really excited about this. I think I’m echoing what the rest of the panel here is saying, but I’m taking this from a more data-centric approach for AI. So my experience, I spent about 20 years in this community, 12 of that doing tactical operations overseas, and then later went back under the guise of intelligence community doing unconventional operations. And all that time, we employed advanced analytics, we wanted to use it at the edge, but today we have the capabilities to start to really bring it to the edge. And so, some of the questions that I wanted to approach today were questions related to data, and how to manage that data so that we can get it to the tip of the spear where it needs to be. So I’m looking forward to this. Thank you very much.
Lisa Costa:
Thank you. And it should be a testament to how short I am that they have had to adjust the mic about five times while I’ve been up here. I think General Thompson might have been up here before me. So my first question is really for Kay. And as you know, we in the Department of Defense have been implementing AI and ML into our systems and our acquisitions for a few years now. In fact, that is exactly how we’re mostly getting AI and ML into our systems. From the 50,000 foot view, what do you see as the primary enablers but also challenges to getting AI and ML right?
Kay Sears:
Right. Thank you for that. I think some of these enablers that I’ll talk about obviously have a challenge to them. It’s kind of both sides of the coin. But it does start with this modeling simulation and ultimately test environment that we are going to create, are creating, are building on. And certainly with the Air Force and AFRL, ensuring that industry and the Air Force are coming together in these environments, that they have the tools, whether it’s AFSIM or some of the industry tools, to really start to build accurate modeling and simulation of AI capabilities. And then, I think we have to take that to test, and we have to test and build those engines again and again. The predictability building in additional complexity, additional processing of inputs, so that we ultimately get to the machine learning aspect of AI.
So I think that that collaborative environment is absolutely critical for us. And I think the Air Force is actually doing a fantastic job in setting that up, inviting industry in, allowing us to bring our platforms, our sensors, and our apps, and start to demonstrate and interact.
We have a virtual Warfare Center where that is where we start to think about the mission that an autonomous with AI enabled system would go try to solve. So understanding those CONOPS is really critical. That’s a critical enabler. What are we solving for? How is this platform going to be used? What is the data that the sensor is going to need to generate in what timeframe? So really understanding the complexity of the problem that we’re trying to solve, that’s how we start to program the AI engines on what data to gather and how to build those. And we do that in a virtual Warfare Center, then we move it in to actually operational software on real platforms, and then we take it and we actually fly it and we start to test it. All of that gathers the data necessary. So I think that’s very key as well.
Open systems. I think, as in your introductory comments, this is a team game. We need everyone. As I’m building right now to autonomous platforms, the MQ-25 and the MQ-28, I want to work with the sensor providers and the payload providers in a very open way. We want to make sure that the vehicle management software is integrated, has some protection, but there’s mission software and apps that have to be brought into that, each of which will have its own kind of AI characteristics. We want to understand what those are and make sure that we’re all talking in the software realm. The digital thread, I think, is a very critical enabler to all of that as well.
I’m just going to throw this out there. Policy. Policy is an enabler. It can also be a major challenge. So as we start to talk about autonomous systems that are making decisions and reacting with input from data that they are getting, not just identification and classification, but actually moving into decision making, the real war fighting tool that it can become, ee are going to have to have policy guidelines around that we all understand and that we can monitor. Leveraging other industries. I think that’s a big enabler as well. How do we leverage the car industry in terms of the AI capabilities that they’re deploying right now. The medical industry. So what can we learn from that?
Ensuring we have a common language when we talk about that. A lot of buzzwords in this environment right now. How do we want to talk about it? I think that’s certainly a big enabler that can also be a challenge. Constant updates from our customers on the threat data. That’s a continuous piece that industry needs. We need to constantly be understanding the threat data and being able to model that. I think the challenges, some specific challenges, go to when you really get into validating non-deterministic behavior, how do we validate that? That’s a new frontier for us. And it’s going to be very important, because the one thing that we have to convey with these AI systems, especially autonomous AI systems, is trust. If you’re a pilot and you have a few of these things on either side of you, you want to be able to trust that you know what those are going to do and they’re going to do it right and they’re not going to cause harm. So that’s very, very important, I think, for that. Adoption. That’s going to be a challenge. I think it’s helped with trust.
Let’s see. And people. I’m just going to throw that out. We’re in a battle every day for the right people with the right skill set. Obviously, AI and machine learning is an area that’s in high demand. We have to be able to attract and hire the right people in the aerospace and defense community within the services as well. So those are just a few enablers and challenges to discuss.
Lisa Costa:
Absolutely. And I absolutely love your comment about the density of war. And this next question is for Nick. And it’s not only the density of war, but it’s the speed of war, and that’s really where AI and ML will have a huge payoff. So for Nick, what role does clean, plentiful, and consistent data have in getting the most out of AI? And what will help achieve faster time to target, additional time on target, additional time to decision making, and higher confidence scoring on decision making?
Nick Toscano:
Yeah, thanks Dr. Costa. And Kay, that was some wonderful points. I really appreciate you talking about the trust in AI and machine learning and bringing that out. That kind of sets up the data piece. I think these questions were wonderful, by the way. You see I have some paper on my lab here because I was iterating over them for a couple days because there’s so much you can go into on some of these questions. But I want to be efficient for you guys on answering this with the limited time that we have.
So the simple answer is, and I think we all know this, is that data’s the lifeblood of AI. I mean, AI is there to reason, to make reason out of data. So how do we handle that and how do we think about that? Well, I asked a counterpoint question is why would you want to work on dirty data? You don’t. As an AI professional and a machine learning engineer, you don’t, because it’s not secure, it’s costly to the organization, it’s not performant. There’s a lot of issues in dealing with that, and you really can’t do enterprise AI on poor data practices.
So it comes down to, really as an organization, we have to think about how do we want to build our data pipelines? What’s the best way to do that? What kind of data management platforms do we want to use within our AI systems? And I’m talking to you about this from an operator perspective as well, having deployed some of these systems down range, having worked on them within National Intelligence. From a user basis, I’ve seen firsthand how poor data management practices can shut down a project or terminate an operation because you don’t have the ability to make the right decisions.
So some of the things I wanted to point out here. Another piece of this question I just want to touch on. We often get wrapped around with what’s called munging data. And we’ve spent about 80% of our time, “statistically” I guess, as machine learning engineers doing that process. So one answer to this question for me is that in terms of having better data pipelines, we also have to make those practices better. And I think that comes to some of the things that Kay is saying in working with industry, looking at our applications and some of the things that we’re bringing into the building, and aligning those resources so that they’ll help us to be more efficient at the data munging or data cleansing and preparation processes.
Let’s see. The last thing on this that I will point out here, and actually Dr. Costa, do you want me to go ahead and answer the second piece of the question on that, or do you want to save that?
Lisa Costa:
Yes, please.
Nick Toscano:
Okay. Oh, wonderful. Okay. So on this piece, I really wanted to give you guys a couple examples of where I think we’re going as an organization. Some the AI tools that we’re adopting as an organization that’s going to help with creating better data and getting faster time to target. So, one of the examples I think is using Managed Data Science Services. These are services that are stood up. It’s click button services that we can use, launch rapidly, but the important thing is we’re not spending time as operators building these environments and managing them. Those are done for us behind the scenes. I’m seeing those things come in at a high level to organizations. They’re providing great benefits. And I think those are things that are going to come into our organization here with air and space, and really improve the processes we’re doing.
Some other things I’ll touch on, and this is something I want to conclude on with today, is leveraging augmented analytics. This is the process of enabling our intelligence analysts, our business analysts, to leverage machine learning applications within their analytic workflows and decision cycles. And what we want to do is not require them to be machine learning engineers, but to give them the ability to leverage those algorithms without having to have a deep statistical or data science or computer engineering background.
Last thing I’ll just throw out there, that I think is a really interesting advancement in AI is called AI services. Really, these have been around for a while. They’re pre-built models, but the interesting thing about them now is we’re getting to the point to where we can operationalize these on a wider scale and deliver them in a manufacturing sense to a defense organization or to our national security organizations to leverage them. And I think the important thing with those, is it’s enabling more pervasive AI services across the organization. It’s enabling more people to leverage complex models and algorithms in the work that they’re doing. A good example would be computer vision or natural language processing as we opened up with at the beginning. I think that’s it.
Lisa Costa:
Absolutely. And that leads to our third question, our next question, for Justin. And it’s really a key question that we struggle with, I think, in the Department of the Air Force in terms of AI and ML. And that is, what are the elements do you need to implement AI and ML that is scalable, sustainable, and successful with the users?
Justin Woulfe:
That’s a great question. So I guess, first step is certainly policy. When we look at the algorithms and things that we develop at Systecon, and certainly many other organizations do as well, making ensure that we can predict the future, make sure we have the right spare part, the right person, the right system available to meet our mission requirements. That’s, of course, bringing together multitudes of traditionally disparate silos of information. And so, as we look at some of the initiatives with Advana and BLADE, things like that that are looking to consolidate and bring this data into a single environment to make it available for these algorithms to actually run. And that’s going to be a big first step.
And then second is, I think, helping to cut down some of those barriers as part of our policy initiatives between industry and the DOD to get industry access to that data set very, very early in the process, so that as they’re designing systems, they’re able to interact a certain way. We’ve got to find a way to get past PDF c-drills being delivered to a program, as we think about delivering logistics product data or reliability and maintainability information, and find a way to get more direct access to those systems, and then get the DOD access on the backside to that OEM data set as well. And I think if we can bring together these traditional silos, we’re going to be very, very successful in being able to not only have autonomous systems operate, but use AI and machine learning to predict outcomes right before we ever even step onto the battle space.
Lisa Costa:
Absolutely. And this question is for all of you, but I have to just set the scene because this is my favorite question. I have science, technology, and research under my portfolio. And part of what we do is we run the space futures program. And what is amazing to me is that if we were to have a 50 person working group, and we were to have met 50 years ago, we would’ve probably put 80 to 90% of the current space environment that exists today in the cone of impossible. Certainly improbable, but much of it would be impossible. And you think about that, and now at the time that technology is moving at such a quick pace,
And the business environment is being driven so much by the commercial enterprises in space. So I’m not going to ask you as hard a question of looking 50 years out, but if you were to look 10 years from now, if you had a crystal ball, what are some of the things that you would imagine that the Department of Air Force would be able to implement in AI/ML that we have not been able to do so today? And you can take your turns.
Justin Woulfe:
I mean, I’m certainly happy to jump on that. So I’ve got a 13 year old and a 10 year old and an eight year old, and I think the eight year old will probably never drive on her own. And so, I think, you’re going to see that across the Air Force where you’re going to have autonomous systems operating alongside of humans. That’s, I would say, almost a guarantee inside of that 30 year window for sure.
And then I think when you take that one step further with the advancements in edge devices and things like that to do on platform analytics, so that we can understand not only from a strategic level what our SAF/SA group does, understanding the probability of mission success and sordid generation rates for the next two, three, four years, you’re going to see that at the wing level before an aircraft ever takes off. They’re going to understand what the probabilistic outcome is on a tail number by tail number basis. You’re going to see in air combat effectiveness on being done like, you should not do this maneuver because, and that’s assuming there’s even a person still in the plane. And so I think you’re going to see more augmented information being presented and being available so that we can actually make better and better decisions looking forward.
Lisa Costa:
Thank you. Okay, Nick?
Nick Toscano:
All right. Thanks, Kay. That was a wonderful answer. Thank you. This was a really good question. So I spent some time the other night trying to use our regression algorithm to get a good answer for you guys, but the confidence score wasn’t high enough so I’m going to throw that out. Thanks for laughing at that joke. Yeah.
Lisa Costa:
Hey, I wanted to borrow the algorithm.
Nick Toscano:
Yeah. So I do want to give you guys, too, a tactical example and a strategic vision type example for this very quickly. My tactical example kind of relates exactly to what we were just talking about, in that very specifically, I think, advances in computer vision are going to do wonders for how we do operations overseas. For example, as a young soldier, I spent a lot of time watching drone feed, and I’m sure we’ve all been there, right? Staying up all night, 3:00 AM in the morning, watching the drone feed fly over, and marking what was important that we saw. That was a mind numbing experience. It was a great experience, but mind numbing. Computer vision can do that for us. We’ve already got great examples of this occurring in computer vision. What I haven’t seen is I haven’t seen us adopt this widely. And that’s going to be a conversation that goes back through some of the stuff we’ve talked about.
That’s going to be a conversation with leadership on what do we want to give AI automation task over? And so do we trust it to look for some of these important targets and recognize some of these important objects? I think we can do that. It’s just a matter of employing it. As a strategic vision, a little bit bigger, I want to talk about augmented AI workforces, and we’ve already been echoing that through conversation. Okay, you brought that up in the very beginning, I believe.
And I think we see evidence of this occurring already through DOD’s ethical AI principles document that’s out there. It’s on the web. There’s five principles that you can all read about. You all probably know about this. But I think that’s opening the gateway for us to create more augmented AI workforces. And what this really is it’s about human to machine teaming to get faster time to target, to be able to make better decisions in the operations sense. And that’s very real. I think we can do that in the near future, and I think that’s going to grow and become a better and better piece of our operations over the next 10 years. I think that’s it. Thank you.
Lisa Costa:
Thank you.
Kay Sears:
Great answers. I’ll build on that, I guess, a little bit. Again, I think in the future fight, I would imagine in 10 years, and I would hope, that we have collaborated as government and industry so tightly that we have the best answer, better than our adversaries, in terms of the balance between human and AI/ML. And that balance gives us an enduring advantage in the fight, a better lethality, better use of our human assets such that we can execute a campaign and ensure our victory. Maybe that’s a little Pollyanna, but that’s my wish in 10 years. I think some of the things that would enable us to do that, we have to get right now, right today, to enable that future.
And I think, again, that’s a very cautious approach to AI/ML in this environment. It’s a balance between the human element, the pilot element, and the unmanned autonomous AI element. I do think some things like neural networks and future technologies that will enable those AI enabled assets to become better decision makers, certainly faster decision makers, and very accurate in terms of their decisions. And I think that’s going to be something that we want to take advantage and deploy in the right ways. So, that’s kind of my vision for 10 years ahead of us.
Lisa Costa:
Thank you. As the panel was speaking, I was reminded of, I was the senior tech advisor to the senior-most SEAL at the time, and I remember talking to him about technology and where the future was going. And he said, “No, absolutely not.” I said, “Well, we’re going to have cameras on your gear. We’re going to have monitors on you. We’re going to know what your heart rate is and things like that.” And he said, “No. First thing we’re going to do is we’re going to rip all that gear off. We don’t need anybody second guessing us and we don’t need any…”
And so I’m thinking, this is maybe 23 years ago, and look how much has changed the fact that we do have persistence there in terms of UAVs, the fact that we do have individual cameras and monitors and being able to intercede in a good way during operations and during ISR applications.
And so, I just wanted to, because I can see a lot of people are standing around on the walls too. Can we just have a show of hands of the Space Force Civilian and Military Guardians? Can you raise your hand? I just want to see how many we’ve got. Okay, everybody look around. Not many. Not many. These people are unicorns. Why are they unicorns? Because when we look at the number of people who are in each service, the Space Force has the fewest and it has the largest AOR.
And so what struck me from the conversation of the panelists is that this construct of having digital assistance, and I think that’s regardless of whether it’s wartime operations, but it’s also about digital assistance. I have an executive officer. I have a front office team, but not everybody has that. So, I think that there is a lot that will happen in the space in terms of just being able to have AI assistance that everyone is able to take advantage of, and to be able to actually build low code, no code solutions themselves, and just present an answer as opposed to…
And I think I read, this was many years ago by the way, that Amazon had over 10,000 engineers working on voice interfaces. So imagine, I mean, we barely have that. We don’t even have that in military in the Space Force. So I think that it just indicates the critical partnership that the Department of the Air Force and the Department of Defense will have to rely on, in terms of it’s not just these point presence partnerships, but it’s these partnerships that will endure and will gain strength over time.
Kay Sears:
Just another comment on that.
Lisa Costa:
Yes?
Kay Sears:
Because I think you’re hitting on a way, again, to build trust in future AI when you talk about decision aids, because as the feedback that we get from the human side of that is fascinating and it really helps us evolve the AI in the right direction. So for example, we’re deploying decision aids for pilots right now. If you think about manned/unmanned teaming there, we’re learning the point at which a pilot might be overwhelmed. I can’t be burdened anymore with controlling this unmanned system. I’ve got fighters coming at me, or whatever it is. That is great information to understand, because then we can take that and we can say, “Okay, here is the human element of a point where we really need more AI, because now this system is going to be dropped. It’s not tethered anymore. It’s got to go fly on its own. It’s got to go continue a mission.” So I really believe the decision aid piece is a great way to get more feedback on how to point the AI in the right direction.
Lisa Costa:
Absolutely. I’m going to do a quick speed round, 30 seconds for each panelist, on a couple of words that you would use to describe what is the key to finding the right partners for exploring AI and ML for the best outcome, based on your experience?
Justin Woulfe:
Well, I think Kay used a great set of words, “the crawl, walk, run approach.” And so I think we can talk about things, we can generate requirements documents, we can try to boil the ocean, so to speak. I think it’s better to start with a limited set of information, a limited knowledge base, and then iterate that over time. So I think, in finding partners, it’s finding partners that are willing to work in a very agile way, are willing to learn, are willing to use whatever they learn through that process to continue to get better and actually go prove what they’re claiming, what they’re saying that they can do. But in a very iterative way, rather than trying to gather everything together all at once and then dump a waterfall approach out, and that’s sort of doomed to fail, I suppose.
Lisa Costa:
Thank you. Nick?
Nick Toscano:
Yeah, thank you. So something that I often say is AI is not a transactional thing. So what I’m saying here is, let’s build consultative relationships around AI problems. So between defense, national security, air, space, and industry, we need to build consultative relationships that allow us to understand these problems, interpret the data that you’re working with, and then engineer complex solutions around them that can be reproducible and repeatable. So what I would say is, move from the thought of this is a transactional activity to a consultative activity with your partners.
Kay Sears:
That’s great comment. I would say that the power of AI is in the data. And so we shouldn’t think of it as a proprietary thing. We should think about it in collaborative environments where we can build the engine, the data. We can repeat and challenge and make that really the center point of what’s going to prove out to be ultimately how we leverage AI, how we get the outcomes that we want. And so whether you’re a platform provider, a software provider, a sensor, or a payload, we all have to come together to build that AI engine, because the power of it is in all of our data that we can create together.
Lisa Costa:
Absolutely. And I know that we are standing between you and your lunch, so that is a critical point that I don’t need to be reminded of. But Justin, Kay, Nick, thank you so much for your expertise and your time today.
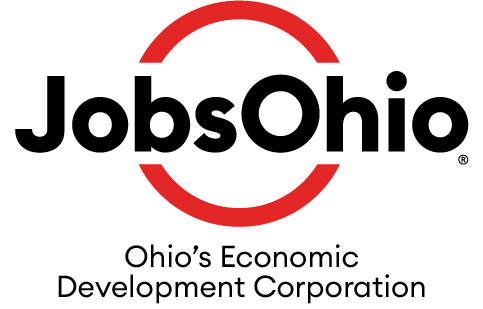